The trilogy concludes.
This is the last of three articles about random numbers in Java programs. The series covers the following topics and also serves as an introduction to the random numbers package of the Uncommons Maths library:
- True Random Numbers and Pseudorandom Numbers
- Statistical Quality (making sure the dice are not loaded)
- Performance (because sometimes you really do need half a million random numbers a second)
- Different kinds of randomness (because not everything can be modelled by tossing a coin)
- Degrees of Freedom (is every possible outcome actually possible?)
- Security (when not losing money depends on nobody knowing what happens next)
Part 3: Seeding
Part 1 of this series discussed different kinds of random number generators (RNGs), highlighted the issues with using the default Java RNGs (java.util.Random and java.security.SecureRandom) and introduced the Uncommons Maths library, which provides three fast, high-quality alternative Java RNGs. Part 2 showed how to generate random numbers from different probability distributions using the classes provided by Uncommons Maths.
One aspect of pseudorandom number generators (PRNGs) that we glossed over in part 1 is the issue of seeding. In this article, we’ll look at two important issues related to the seeding of PRNGs.
Degrees of Freedom
Suppose we want to shuffle a standard deck of 52 playing cards. The Java API provides an implementation of a very good algorithm for shuffling a list (see Collections.shuffle). It even provides a variant of this method that allows us to specify our own RNG to be used. So shuffling a deck of cards (assuming it is represented as a List) is easy in Java.
Unfortunately, even though the algorithm is a perfect shuffle and we can choose an RNG that exhibits no bias, our shuffling code is still likely to be flawed in one respect. The problem is that the RNG is almost certainly not able to generate every possible outcome at each state transition. There are many orderings of the deck that cannot occur. The RNG has insufficient degrees of freedom. To understand why this is, we need to consider how many ways there are of ordering a deck of 52 cards. I’ll spare you the maths and tell you that the answer is 52! (that’s 52 factorial) or, in longhand, 80,658,175,170,943,878,571,660,636,856,403,766,975,289,505,440,883,277,824,000,000,000,000.
That’s a pretty big number.
Recall from the first article in this series that when we seed a PRNG we get the same sequence of output each time that we use the same initial seed. The maximum number of possible initial states for the PRNG is equal to the number of possible seed values. If each RNG state gives us a different shuffle, the number of different deck orderings we can generate is determined by the range of the RNG seed.
The CellularAutomatonRNG is very fast, but it only uses a 32-bit seed. So using it for shuffling restricts the result of any given shuffle to one of 4,294,967,296 outcomes. That’s 4.3 billion possibilities but it may as well be zero given the number of outcomes that should be possible but aren’t. [Note that a second shuffle may well allow a different set of 4,294,967,296 permutations than the first (so over time a larger number of outcomes are possible) but for any nth shuffle the number of possible outcomes is restricted by the number of starting states.]
We would probably want to avoid using java.util.Random but, if we did use it, we’d give it a 64-bit long as its seed. The algorithm only actually uses 48 of those bits for seeding so that gives us 281,474,976,710,656 possible initial RNG states. 281 trillion is an improvement, but still woefully inadequate.
The MersenneTwisterRNG uses a 64-bit seed. That’s 18,446,744,073,709,551,616 possible outcomes for any given shuffle. Even this huge number is a miserable, tiny, insignificant fraction of one percent of the full set of 52! possibilities that we ought to be able to generate.
SecureRandom may use different algorithms internally, depending on the platform. On Windows, the default is the SHA1PRNG provider, which gives us a 160-bit seed. Ignoring SecureRandom’s slowness, this is getting us closer to where we want to be (if you can call less than one trillionth of one percent of the possible outcomes ‘close’).
One more RNG…
It turns out that we need a seed of at least 226 bits in order to achieve the necessary degrees of freedom. This rules out most PRNGs that you might otherwise consider. There is at least one option however: Uncommons Maths’ AESCounterRNG. The AESCounterRNG is based on the AES block cipher. AES is the Advanced Encryption Standard adopted by the US government. The characteristics of AES that make it a good encryption algorithm also coincidentally make it a good RNG. When we use AES as an RNG, the seed is the encryption key. AES supports 128-bit, 192-bit and 256-bit keys.
Using AESCounterRNG with a 256-bit seed, we have the necessary degrees of freedom so that all 52! orderings are possible outcomes of a shuffle. AESCounterRNG, while still much faster than java.security.SecureRandom, is not quite as fast as the other RNGs we’ve been looking at. It does however have other advantages that we shall see later.
Note: Using encryption keys longer than 128 bits in Java requires that you obtain and install the unlimited strength cryptography policy files from Sun.
Security
Before you rush off to set up your new online casino, there’s one further issue to be considered. Remember that pseudorandom number generators are deterministic. That means that if we know which PRNG algorithm is in use and we know what seed was used, then we can predict with absolute certainty every single value that the RNG will ever produce. No problem for non-sensitive applications, but if people are gambling on the outcomes, you’d better be certain that they don’t have this kind of inside information.
Forget about seeding your RNG from the system clock – your seed data needs to be random itself or it is vulnerable (see How We Learned to Cheat at Online Poker: A Study in Software Security for a real world account of how RNG weaknesses can be exploited in online poker). Even if an attacker doesn’t know the seed, with many algorithms it can be reversed engineered by observing the RNG’s output and applying a little computing power.
To address these security concerns, the first step is to pick a PRNG implementation that is not susceptible to reverse-engineering. That brings us back to the AESCounterRNG
. Being based on AES means that reverse-engineering the seed would involve cracking the cipher. Good luck doing that in a timely manner.
Disclaimer: You should not rely on the AESCounterRNG to address all of your security concerns. A fuller understanding of the issues can be gained by reading Ferguson and Schneier’s Practical Cryptography – particularly the description of their Fortuna PRNG design.
So where do we get the seed data from? We have a bit of a bootstrapping problem. In order to generate good quality, unpredictable random numbers we first need some good quality, unpredictable random data. By default, all of the Uncommons Maths RNGs seed themselves from /dev/random
on Unix systems. On Windows systems seed data will be download from random.org and if neither of those strategies is succesful (perhaps the SecurityManager prevents file or network access) then it will fall back on the seeding strategy provided by SecureRandom.generateSeed
. However, seeding strategies are pluggable so that you can provide your own. One possible strategy is to use a true hardware RNG to bootstrap the faster PRNG that will generate the actual output.
The End
And that’s pretty much everything I can tell you about using random number generators in Java. If you have any feedback on Uncommons Maths, please use the issue tracker for bugs and suggestions. If you want to know more about pseudorandom number generators, the following books cover the theoretical and practical issues in much more depth, and much more precisely, than I have done.
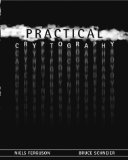